Traditional vs. AI Video Surveillance: A Comparative Insight
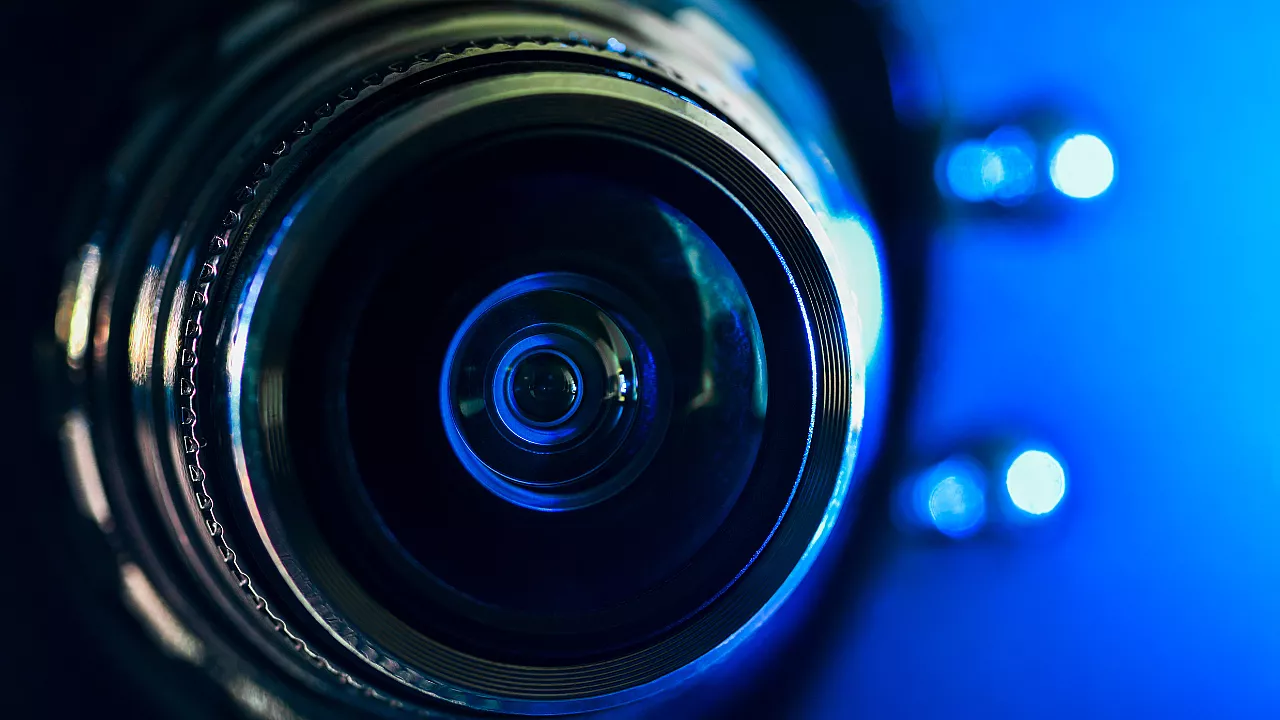
The transition from standard video monitoring to AI-enhanced surveillance has transformed our approach to security. Previously, video surveillance was mostly used to record events, which required someone to review the footage, especially during incidents. But it wasn't feasible to constantly monitor millions of cameras. This resulted in a lot of unwatched footage and missed incidents.
Now, with AI in the mix, these systems do more than just record. They can interpret, predict, and react to situations as they unfold. They handle massive amounts of video data with remarkable accuracy, detecting patterns and identifying threats. This isn't just an upgrade; it's a game-changer for our safety.
Traditional Video Surveillance Explained
Video surveillance, which includes systems like Closed-Circuit Television (CCTV), has been a mainstay in security for years. These systems have protected businesses, homes, and public spaces by preventing crime and recording events.
A quick look back:
1940s: CCTV begins, first used by the German military during WWII.
1950s: CCTV expands in the U.S., particularly in places like banks.
1960s: Cameras appear in public spaces, such as at New York's Olean High School in 1968.
1970s: The Video Cassette Recorder (VCR) was introduced, allowing video footage to be recorded and saved.
1980s: Multiplexing technology was introduced, enabling a single VCR tape to hold multiple camera feeds.
1990s: Digital tech brought timestamped videos, color cameras, and Digital Video Recorders (VRs).
2000s: Cameras begin to transition to digital and use the internet for additional functionality.
The main parts of traditional video systems are:
Cameras: Capturing footage. Old ones were black-and-white; new ones are color and can zoom.
Monitors: Displaying live or saved video.
Recording Devices: Saving videos. Started with tapes, now they use DVRs and Network Video Recorders.
Switchers: Displaying multiple camera feeds on one screen.
Cables/Connectors: Connecting everything together.
The advantages of video surveillance are multifaceted. The mere presence of cameras often serves as a deterrent to potential criminal activities, reinforcing the importance of security in various environments. Beyond this preventive role, the footage captured by CCTV systems holds significant value in legal proceedings, providing tangible evidence when needed.
It’s time to dive deeper into the workflow of these systems.
How Traditional Video Surveillance Works
Traditional video surveillance, or CCTV, uses cameras to capture and transmit video to a central monitor, keeping the feed private. Here's how it works:
Camera Installation: Selecting and mounting cameras in strategic locations to meet specific needs. Using cables to connect them.
Video Transmission: Cameras send video to recording devices, either analog or digitally.
Recording: DVRs or Network Video Recorders save the footage. The length of time depends on the size of the storage unit and the needs of the user.
Viewing: Watching live or saved videos on monitors. Some systems enable remote monitoring.
Playback/Search: Users can search for specific footage based on date, time, or events.
Backup/Export: Transferring important footage to another device for sharing or longer storage.
Maintenance: Regular checks and cleaning. Making sure there's enough storage and everything's working. Implementing necessary software or firmware updates.
Security: Integrating with alarms and protecting footage with encryption and strong passwords.
Though technology has improved, humans remain vital in traditional systems. They install, maintain, monitor, and make decisions based on the footage.
While these systems have worked well for years, they have limits. As security needs grow, we see these systems' constraints clearer than ever.
Limitations of Traditional Surveillance Systems
While applications like crime prevention and traffic monitoring have benefited from traditional CCTV-based surveillance, the technology has a number of limitations:
Field of View: Traditional cameras may have blind spots unless equipped with pan-tilt-zoom (PTZ) features.
Resolution: Older systems might lack high resolution, reducing clarity.
Storage: Limited local storage can be susceptible to tampering.
Analytics: Lack of advanced video analytics requires manual review.
Vulnerabilities: Physical infrastructure can be tampered or damaged.
Lighting: Performance in low light can be suboptimal without infrared.
Cost & Maintenance: Setup and upkeep can be expensive and time-consuming.
Privacy: Without smart analytics, systems can be invasive.
Access & Integration: Limited remote access and integration with new tech.
Latency & Environment: Analog broadcasting might be slow, and equipment may not withstand all conditions.
Volume & Redundancy: Massive footage amounts, much of which may be redundant.
Human Error: Monitoring can result in missed events, false alarms, biases, and inconsistencies.
Despite these drawbacks, traditional surveillance systems have been an important tool in maintaining security in a variety of settings. Many of these limitations, however, are being addressed by newer technologies, paving the way for more efficient, scalable, and intelligent surveillance solutions.
Modern Security Solutions and the Need for Innovation
Video surveillance has evolved significantly, thanks to recent technological advancements, and has reached new heights. Innovation is now crucial to tackle evolving threats and heightened security needs:
High Definition: Modern cameras provide clearer, high-resolution images.
Wide Dynamic Range (WDR): Cameras handle varied lighting conditions for clear footage.
AI & Machine Learning: Real-time footage analysis, including facial recognition, object detection, and behavior analysis.
Edge Computing: Cameras with built-in processing capabilities enhance efficiency.
Cloud Storage: Integrated cloud solutions allow easier data access and backup.
360-degree View: Comprehensive coverage with no blind spots.
Thermal Imaging: Detection of heat signatures, ideal for nighttime.
Integrated IoT: Connectivity with other security features.
These innovations stem from the need to overcome traditional surveillance limitations. Implementing AI-enhanced systems is crucial for effective, modern surveillance across diverse contexts.
Introduction to AI-Boosted Video Surveillance
In an era of data overload, AI is revolutionizing surveillance. Through AI, cameras can sift through vast footage, identifying key events or anomalies with speed and precision, making spaces more intelligent and secure.
The Power of AI in Surveillance
AI's capacity to rapidly process extensive data, detect hidden patterns, and continually learn and adapt is transformative. It bridges the divide between raw data and actionable insights, reshaping industries and expanding possibilities:
Real-time processing of enormous data, ensuring swift issue detection.
Pattern recognition to identify abnormal behaviors amidst usual activities.
Advanced algorithms that multitask, monitoring several video feeds simultaneously.
Abilities like facial/object recognition, and behavioral analysis.
Integration with digital systems for comprehensive surveillance.
Adaptive learning, allowing systems to refine their accuracy over time.
Scalability, enabling consistent monitoring across vast areas.
Long-term cost-efficiency, reducing the need for human oversight.
As we explore the profound impact of AI in surveillance, it's vital to grasp the fundamental technologies enabling this fusion. Let's now delve into the core technologies powering AI-enhanced surveillance and how they breathe life into this intelligence.
Key Technologies Enabling AI-Boosted Surveillance
AI-driven surveillance leverages several key technologies to augment traditional systems, merging hardware capabilities with advanced software:
- Deep Learning & Neural Networks:
Convolutional Neural Networks (CNNs): Image classification and object detection in videos.
Recurrent Neural Networks (RNNs): Analyzing time-sequential patterns in videos.
Generative Adversarial Networks (GANs): Enhancing images by refining resolution or reconstructing obscured parts.
Transfer Learning: Fine-tunes models for specific tasks, conserving computational resources.
Edge Computing: Processes data near its source using chips like NVIDIA’s Jetson or Google’s Edge TPU.
Computer Vision: Alongside deep learning, traditional algorithms like HOG (Histogram of Oriented Gradients) and SIFT (Scale-Invariant Feature Transform) remain crucial.
Sensor Fusion: Combines multiple sensor data for a fuller understanding of environments.
Data Annotation Tools: Essential for training precise models. Semi-automated tools expedite dataset annotations.
Cloud Infrastructure: Platforms like AWS and Google Cloud offer computation and storage for surveillance operations.
Hardware Accelerators: GPUs (Graphical Processing Units), TPUs (Tensor Processing Units), and FPGAs (Field-Programmable Gate Arrays) enhance AI computations for real-time processing.
Embedded Systems: Efficient, compact systems that allow local data processing without constant external connections.
Data Privacy Tools: Techniques like Differential Privacy and Homomorphic Encryption ensure data privacy.
Quantum Computing: Holds potential in encryption and data analysis, though still emerging.
Big Data Solutions: Data Lakes and analytics tools manage the vast data from surveillance systems.
AutoML: Automates model selection and tuning, allowing systems to swiftly adjust to new data.
Together, these technologies empower modern surveillance to predict, detect, and analyze with unparalleled precision.
Machine Learning Algorithms and Automation
Machine learning (ML), a branch of artificial intelligence, uses statistical methods to let computers learn from data. Automation refers to processes done with minimal human involvement. Together, they create adaptable, self-sustaining systems.
ML Algorithms Overview:
Supervised Learning: Predicts using labeled training data. Examples include Linear Regression, Neural Networks, and Decision Trees.
Unsupervised Learning: Learns from unlabeled data, with techniques like Clustering and Association.
Reinforcement Learning: An agent maximizes rewards through actions. Methods include Q-learning.
Semi-supervised & Transfer Learning: Utilize both labeled and unlabeled data or use pre-trained models for related tasks.
Key Concepts:
Data Processing Pipelines: Systems like Apache Kafka manage data flow automatically across stages, from collection to prediction.
Model Training Automation: Tools such as Google's AutoML streamline model selection, feature engineering, and tuning.
CI/CD for ML: Jenkins and similar tools automate training, testing, and deployment for consistent model performance.
Implications of ML Automation:
Scalability: Technologies like Kubernetes ensure systems adapt as data or needs change.
Efficiency & Reliability: Automation optimizes resource use and monitors ML system health, acting on any anomalies.
Version Control: Automation maintains data and model change histories, crucial for auditing.
Feedback Loops in ML:
Systems continuously learn from new data.
Real-time adjustments and self-correction from mistakes enhance model accuracy.
Technological advancements in machine learning have revolutionized surveillance. In the past, surveillance was manual and resource-intensive, dependent on human vigilance and physical recording. Today, AI-powered surveillance offers increased efficiency, accuracy, and scalability. It's essential to compare traditional and AI-based surveillance to understand their functional differences, efficiency, and societal implications.
Comparing Traditional and AI Video Surveillance
At NSoft Vision, we've dedicated our efforts to advancing the realm of video surveillance. While we've seen firsthand the transition from traditional systems to AI-boosted solutions, understanding the differences between the two can provide valuable insights. This comparison, drawn from our experiences and insights from our clients, aims to shed light on the evolution of video surveillance.
1. Monitoring & Detection
Traditional: Manual monitoring is prone to human errors. Over long shifts, security personnel might miss subtle but crucial incidents. Historically, there have been challenges related to fatigue and oversights.
AI-Boosted: AI systems can consistently scan footage 24/7. They can identify unusual patterns or potential threats without getting tired, offering a constant vigilance that human teams might struggle with.
2. Data Processing & Storage
Traditional: Storage primarily relied on local setups. This often led to limitations in the amount of footage stored and sometimes slow retrieval of specific video segments.
AI-Boosted: Cloud storage integration and AI tagging have made it easier for users to store vast amounts of footage and retrieve specific segments quickly.
3. Efficiency & Scalability
Traditional: Expanding a security setup often meant linearly increasing costs, as more personnel would be needed to monitor additional cameras.
AI-Boosted: AI systems allow for more cameras to be added without a proportional increase in monitoring personnel, since the AI can handle multiple feeds simultaneously.
4. Cost Implications
Traditional: Continuous costs from maintenance and manpower were a concern for many organizations, especially when considering expanding the system.
AI-Boosted: Although there's a potentially higher initial setup cost, over time, organizations can see savings due to reduced manual monitoring needs and streamlined maintenance.
5. Precision & Accuracy
Traditional: Relies heavily on the experience and expertise of the operator. The effectiveness can vary depending on the individual's alertness and judgment.
AI-Boosted: AI systems maintain a consistent level of accuracy, reducing human errors and biases. They objectively analyze footage based on algorithms.
6. Flexibility & Integration
Traditional: Earlier systems had limitations when it came to integrating with newer technologies or devices.
AI-Boosted: Modern AI solutions are designed with integration in mind. They often work seamlessly with other IoT devices and technological platforms.
7. Response Time
Traditional: The speed of response depends on human reaction times. Delays can sometimes occur if an incident goes unnoticed.
AI-Boosted: AI systems can trigger automated alerts the moment they detect anomalies, potentially reducing response times during critical situations.
8. Privacy Concerns
Traditional: Continuous recording sometimes raised privacy concerns, especially in areas where individuals felt constantly watched.
AI-Boosted: AI systems, with features like facial blurring or selective recording, can help address some of these privacy concerns while still maintaining security.
From experience, it's evident that both traditional and AI-boosted surveillance systems have their strengths and limitations. As technology continues to evolve, so will the landscape of surveillance, and it's essential to stay informed to make the best choices for security needs.